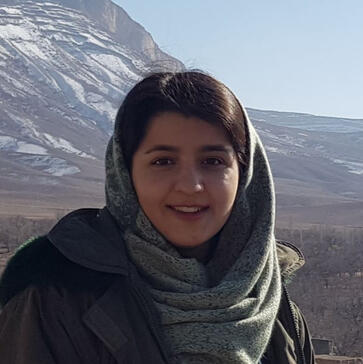
Setareh Soltanieh
Graduate Researcher
About
Welcome to my page
I am Setareh Soltanieh, a graduate researcher in MITHRIL at Queen's university under supervision of Dr. Matthew Pan. Previously, I was a machine vision researcher at Avir Artificial Intelligence Center under the supervision of Dr. Akhaee.My research interests broadly span Augmented Reality (AR), Virtual Reality (VR), computer vision and machine learning.
I completed my B.A.Sc degree in Electrical and Computer Engineering in 2021 in the University of Tehran.
Contact
Please reach out and let me know if I can be of assistance with your projects! :)
[email protected]
Soltanieh S., Toosi R., Akhaee M., "Digital Image Watermarking Employing Sample Volume Quantization", Elsevier Expert Systems with Applications
My life is always separated into two parts. One is Programming and Mathematics, and the other part is Painting.
For as long as I can remember, I have been adored for my talents in both mathematics and drawing.
Here are some of my recent arts.
Enjoy! :D
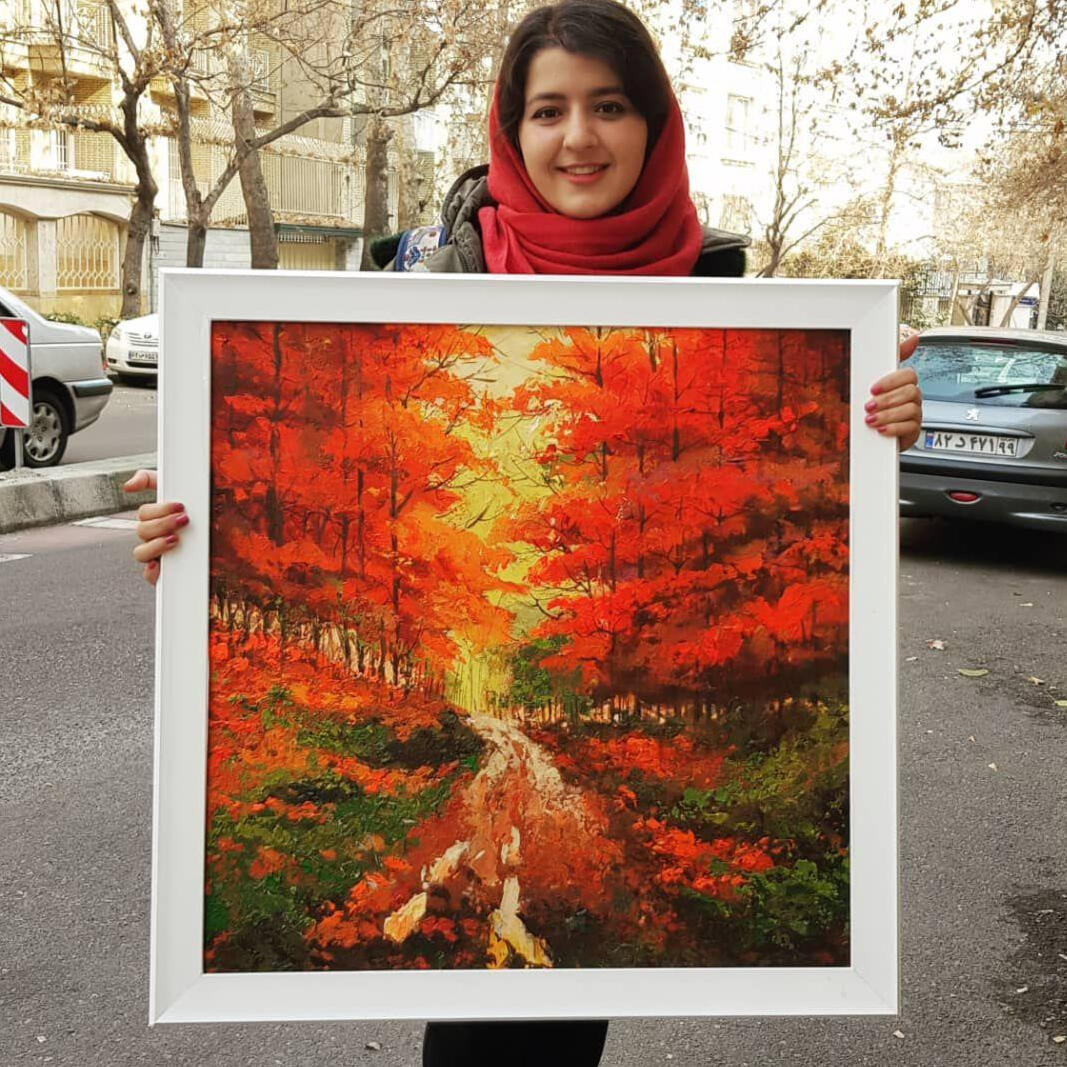
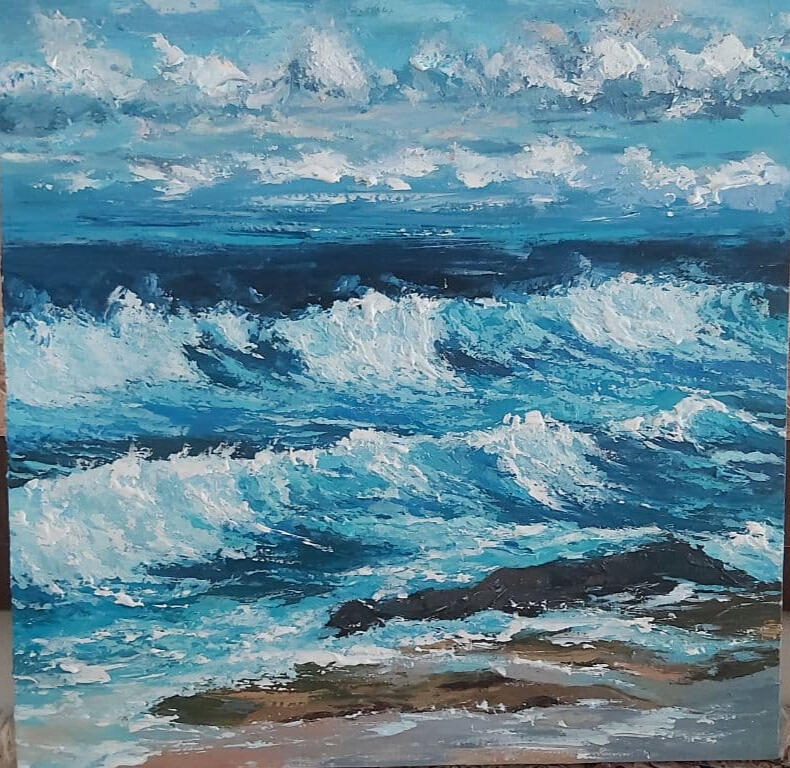
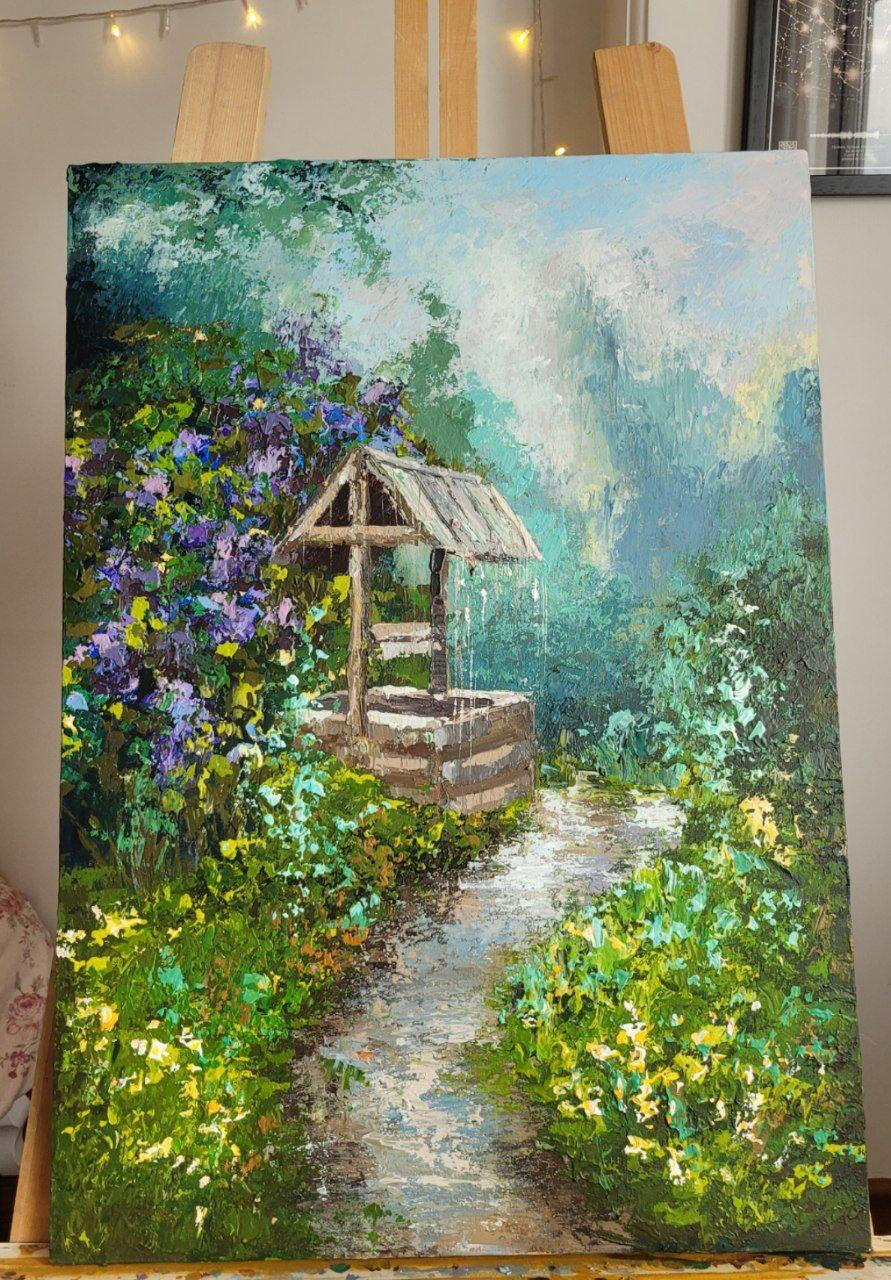
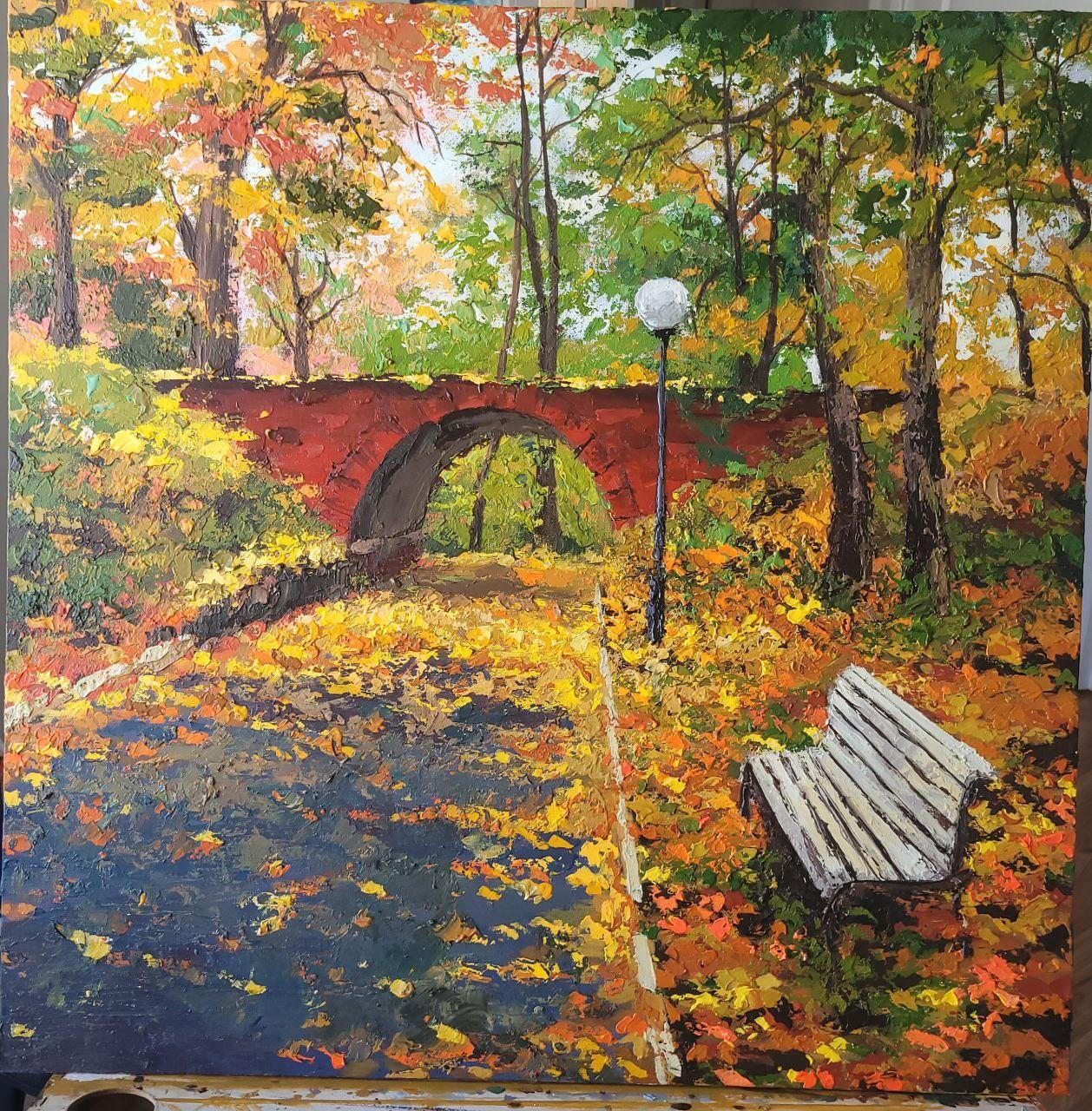
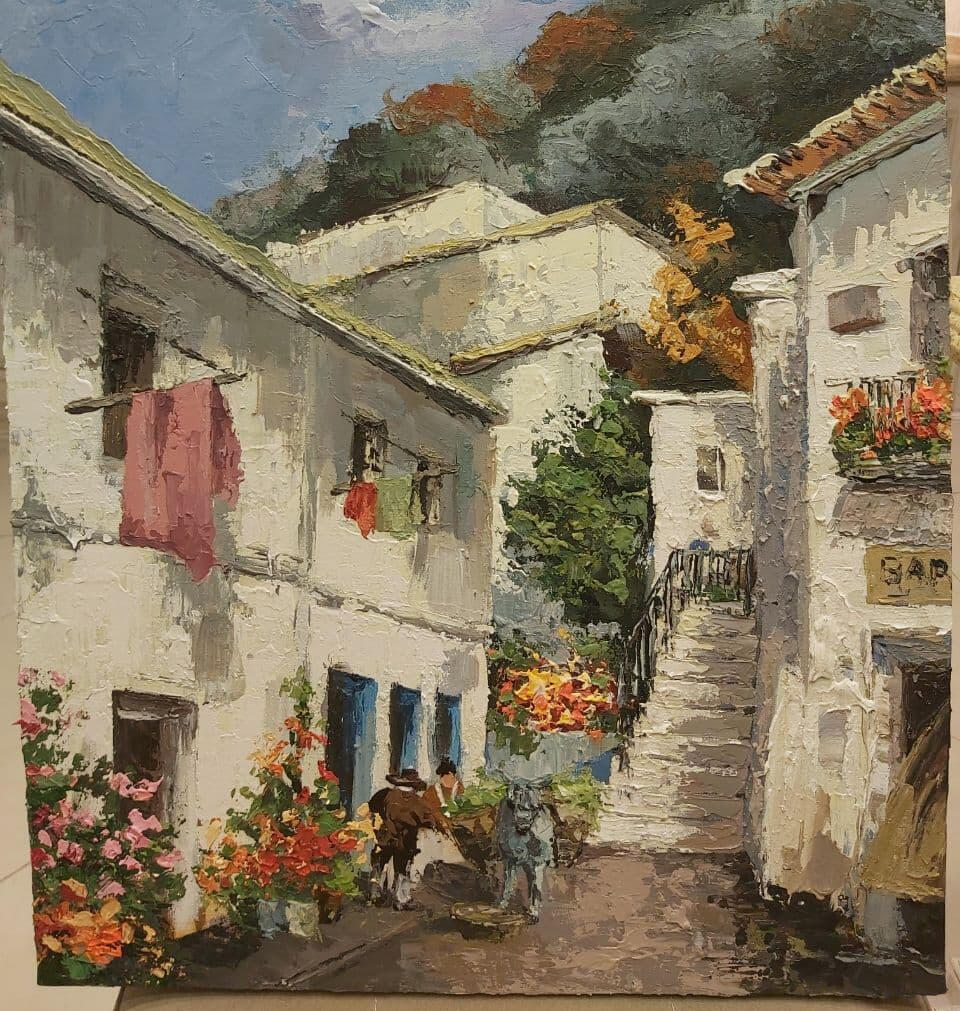
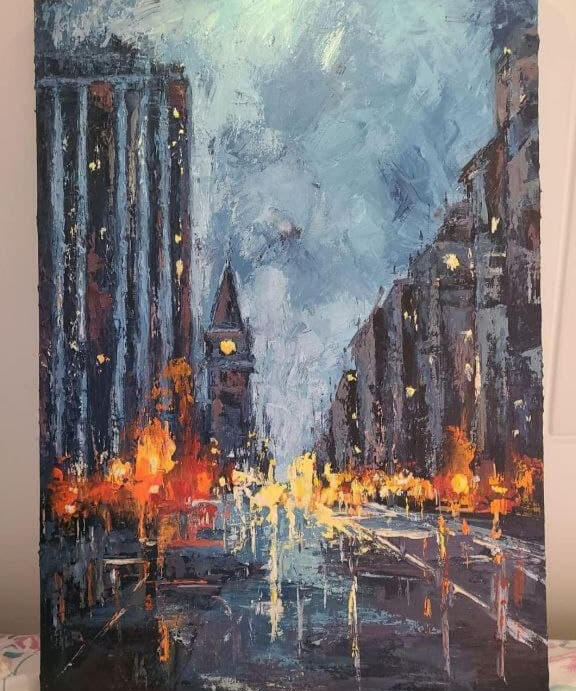
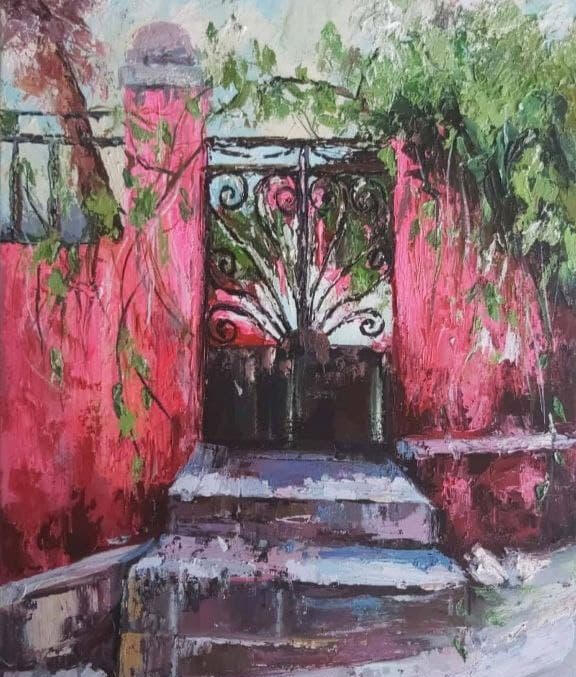
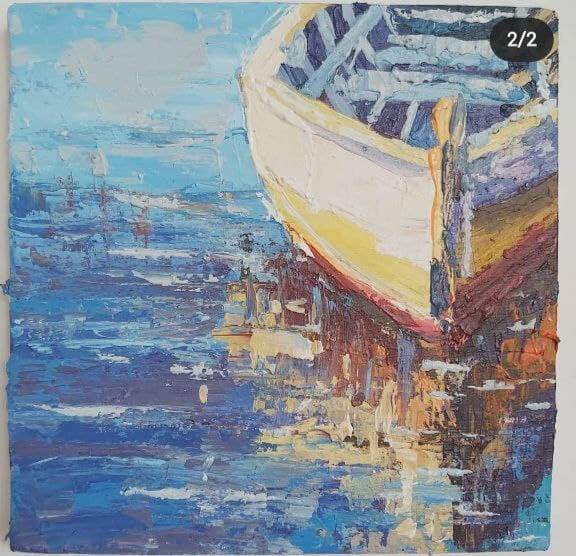
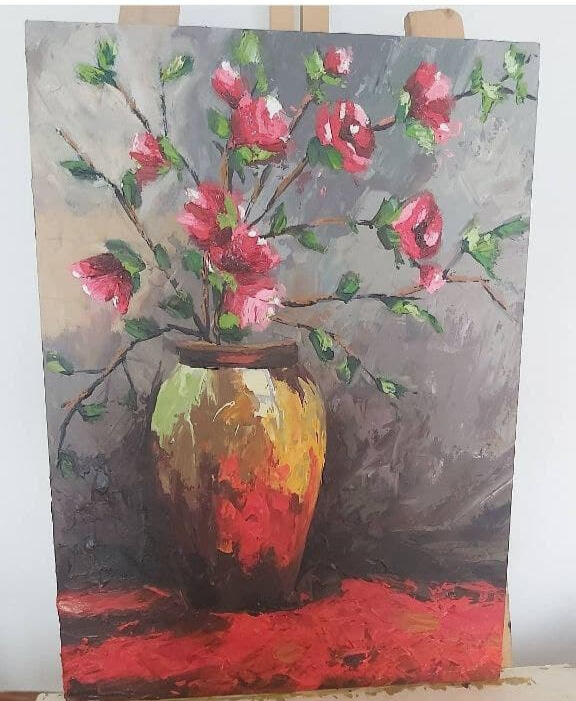
In this project, a watermarking scheme using sample volume quantization is proposed.
The host signal is first transformed to the frequency domain using discrete wavelet transform (DWT). Then we form a polyhedral from the transformed values. The watermarked samples are calculated by quantizing the volume of the polyhedral to specific values which depends on the input bit.
For minimizing the distortion, the watermarked samples are acquired as close as possible to the host samples while maintaining the quantized volume fixed. An optimization problem is solved using Lagrange multiplayer method. Since the obtained equation is quite complex, we use gradient descend method. Finally, a maximum likelihood detector is designed for watermark extraction at the receiver side.